Monte Carlo Simulation – Don't Build a Bad House
Two takeaways for lawyers about Monte Carlo simulations, from finance columnist Michael McDonald.
Monte Carlo simulation is a technique that is widely used in both the financial and legal industries. Yet many people are using it incorrectly and don’t understand the limitations of Monte Carlo simulations. In brief, a Monte Carlo simulation is a tool like a hammer or a wrench. And while the technique is useful many people use it improperly, and come to a bad conclusion just as one can build a faulty house by using a good hammer incorrectly.
Monte Carlo simulations are essentially experiments. They test what would happen in a situation over and over again so that one can see the probability of a particular event occurring.
For example, a financial professional might want to know what their returns will be on a portfolio of stocks – a Monte Carlo simulation creates a set of hypothetical price paths that tell the user what the expected future returns are on the stock on average. This expected future return is usually based on 100, 1,000, or 10,000 hypothetical simulations – in other words, if you were to go through the process of owning the portfolio 100 times in exactly the same conditions, what would your returns be? Monte Carlo gives us the answer.

AI Presents Both Opportunities And Risks For Lawyers. Are You Prepared?
Monte Carlo simulations are similarly useful for attorneys in a variety of settings. For example, an attorney could use such a simulation to determine the expected outcome in a case given various parameters, or to determine the odds of an injury in a medical setting, or to aid in determining fees for customers.
Monte Carlo simulations are also used by a few litigation finance firms to project expected returns for their portfolio. They should be used much more widely in that field, but many firms lack the expertise to set them up properly, or believe that the results are not worth the upfront cost of setting up the system. (The latter is a mistake – having robust risk management and measurement tools in place can dramatically lower cost of capital for a funder.)
Still despite all of the benefits of Monte Carlo simulations, they are often misused. In particular, the key with a Monte Carlo simulation is that the model behind the scenes is valid.
For example, if we are trying to model what will happen to a portfolio of stocks, we need to make sure we are using the appropriate mathematical formulas to describe stock price movement. I can write a formula that says stocks go up or down $1 each day with a 50% probability. That’s an incorrect formula, but I can easily build a Monte Carlo simulation around it nonetheless.
Sponsored

AI Presents Both Opportunities And Risks For Lawyers. Are You Prepared?

Ranking The Law Firms Lawyers Love
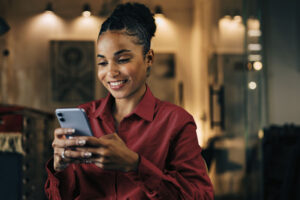
Law Firm Business Development Is More Than Relationship Building
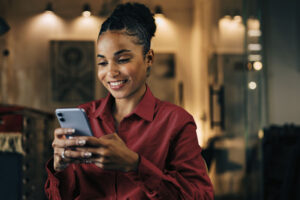
Law Firm Business Development Is More Than Relationship Building
The problem that occurs in both the finance field and the legal field is that too many people hear “Monte Carlo simulation” and become seduced into believing that the results are valid. Those results are only as good as the formula that the simulation is based on.
In many cases, people used canned software to run Monte Carlo simulations such as Crystal Ball or @Risk. While these tools are easy to use, they are also easy to misuse. Because these types of software packages come prebuilt, users often don’t understand what the formula is that drives their results, and in my experience the output is generally questionable as a result.
Is there a better way forward?
The answer is “Yes.”
There are two takeaways that should be kept in mind in the future.
Sponsored
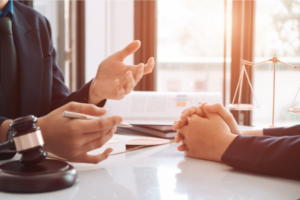
Curbing Client And Talent Loss With Productivity Tech

Luxury, Lies, And A $10 Million Embezzlement
First, professionals in a wide range of industries, from finance, legal, medical, and beyond, should realize that Monte Carlo simulations are useful when used properly. Too many people dismiss the simulations as too complex or time-consuming.
Second, when using a Monte Carlo simulation, always go deeper than the basics. Don’t just ask how many times the simulation will run or what the results mean. Instead, users should ask what the basis for the results is. Where did the formula that the simulation relies on come from? If the simulation is built around historical data, does that historical data accurately represent the future? How does the data or formula take into account the possibility of outliers or tail events?
These questions take time to ask and answer, but they will produce much better results at the end of the day, and a much better outcome for users.
Earlier: Finance And Law: The Pros And Cons Of Monte Carlo Simulations In Valuation
Michael McDonald is an assistant professor of finance at Fairfield University in Connecticut. He holds a PhD in finance. Michael consults extensively with organizations ranging from Fortune 500 companies to start-up businesses on financial matters through Morning Investments Consulting. Michael has served as an expert witness in legal disputes, and is an arbitrator with the Financial Industry National Regulatory Authority (FINRA). Michael can be reached at [email protected].