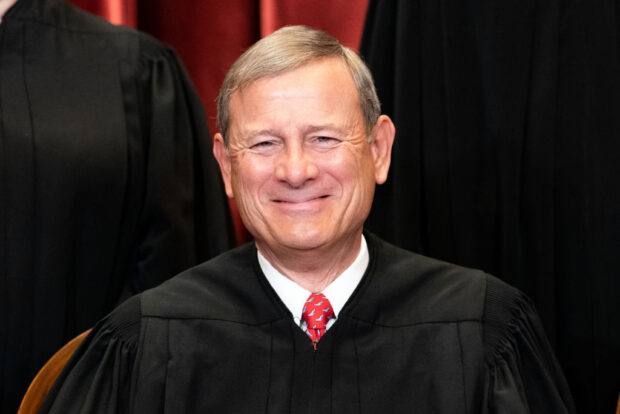
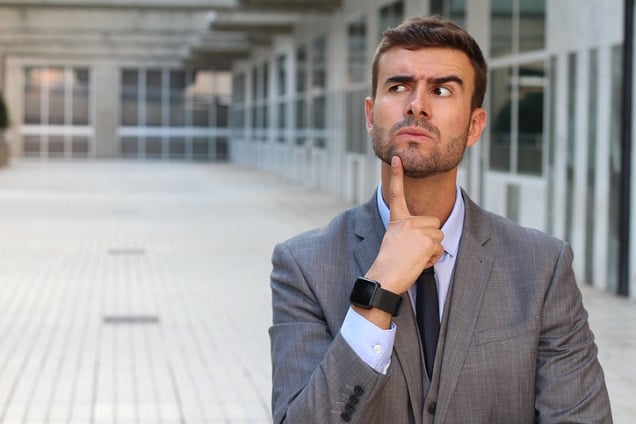
A Footnote (4) To History
What kind of product would a Carolene Products produce if a Carolene Products could produce products?
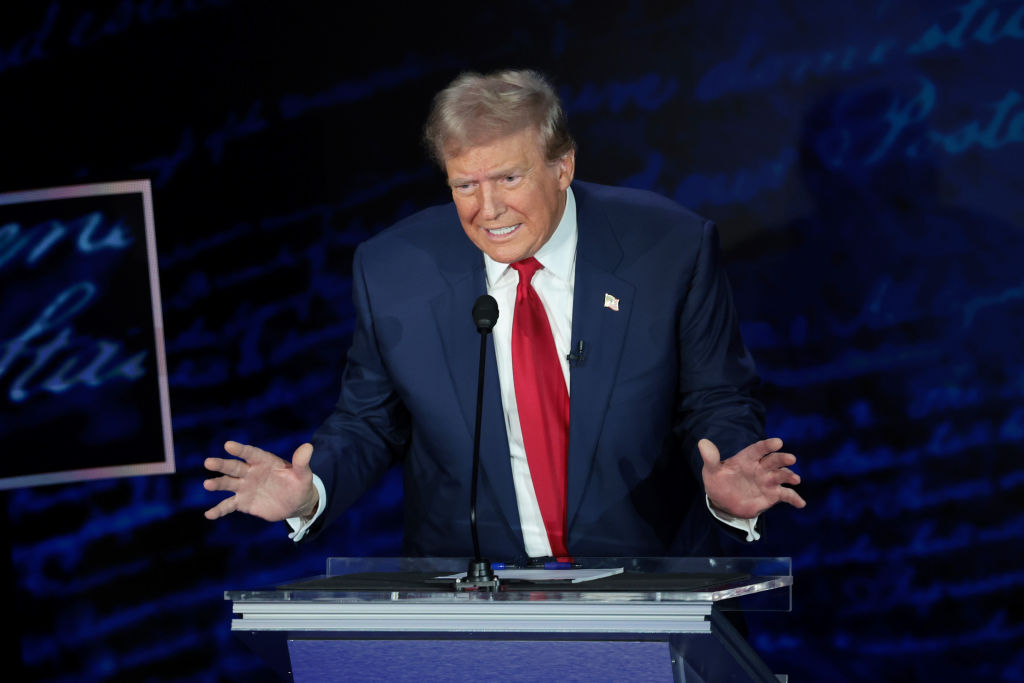
Trump Administration Places Thumb On Negotiation Scale, Decides Harvard Didn’t Do Enough To Fight Antisemitism
Negotiations are underway...
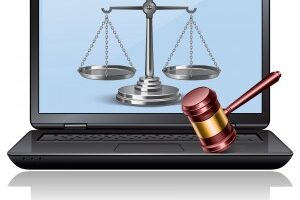
You’re Selling Legal Tech All Wrong: Chad Aboud’s Playbook For Getting Buy-In
One of the biggest mistakes in-house lawyers make is framing legal tech as something that solves legal problems.
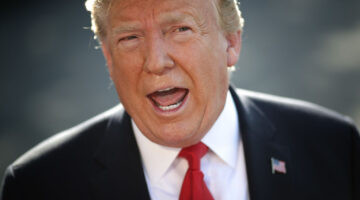
Biglaw Firm Made A Big Mistake By Signing This Deal With Trump
The firm isn't just losing lawyers, but respect within the legal industry.
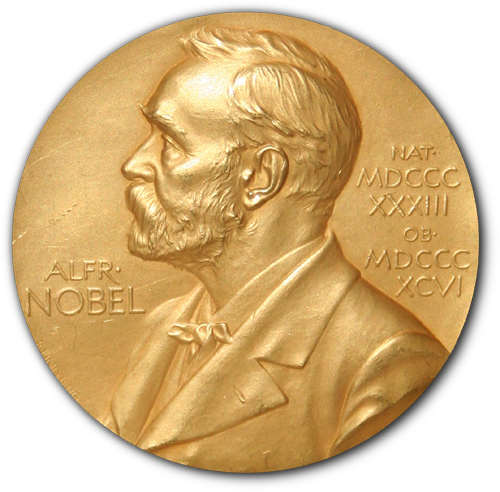
Does Trump In Fact Deserve The Nobel Peace Prize?
At first glance, it appears that Trump has pulled off a near-miracle.